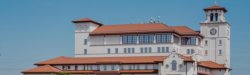
View Profile Page

Aparna Varde
Associate Professor, School of Computing, College of Science and Mathematics
- Office:
- Center for Computing and Information Science 227A
- Email:
- vardea@montclair.edu
- Phone:
- 973-655-4292
- Degrees:
- BE, University of Bombay
- MS, Worcester Polytechnic Institute
- PhD, Worcester Polytechnic Institute
- vCard:
- Download vCard
Profile
Dr. Aparna Varde is a tenured Associate Professor in the School of Computing (SoC) at Montclair State University (MSU), NJ, USA. She is also an Associate Director of the Clean Energy and Sustainability Analytics Research Center (CESAC) at MSU. She has served as the Inaugural Associate Director for Graduate Studies and Research in SoC at MSU for a year. Dr. Varde has been a visiting researcher at Max Planck Institute for Informatics, Saarbrucken, Germany multiple times. Her work spans Artificial Intelligence, Machine Learning, Data Mining, Databases, Environmental Computing, and Computational Linguistics. Her honors include 9 best paper awards at IEEE conferences & other venues. She is Doctoral Faculty in the PhD Program in Environmental Science and Management at Montclair. Dr. Varde has over 150 publications (journals, conferences, book chapters, edited volumes) by IEEE, ACM, AAAI, Springer etc. Her current research projects entail Commonsense Knowledge, Explainable AI, Smart Cities, Geo-informatics, Robotics, and Text Mining. She has been a dissertation advisor, committee member, and mentor for PhD students at Montclair State University (including a Fulbright Scholar) and an external examiner / referee for PhD students worldwide (including Queensland University of Technology, Australia). Dr. Varde has been a panelist for NSF, PC member at conferences, and reviewer / editorial board member for journals, by IEEE, ACM, Elsevier etc. Her research is supported by grants from organizations such as PSE&G, NSF and NOAA. She has generated more than 2 million dollars of external funding in various capacities. Dr. Varde is classified as an outstanding researcher by the Citizenship and Immigration Services, USA.
Specialization
Artificial Intelligence - Commonsense Knowledge, Explainable AI, Smart Cities, App Development with HCI, Implicit Requirements in Software Engineering, Collaborative Robotics, Expert Systems
Machine Learning and Data Mining - Predictive Analytics, Decision Support Systems, Domain-Specific Knowledge Discovery, Text Mining and NLP
Database Systems - Big Data Management, Cloud Computing, Web Databases, XML and DSML
Environmental Computing - Clean Energy, Green IT, Urban Policy, Geo-informatics, Sustainability, Drone Data Analysis
Links
Montclair State University does not endorse the views or opinions expressed in a faculty member's webpage or website. Consistent with the principles of academic freedom, the content provided is that of the author and does not express the opinions or views of Montclair State University.
- Aparna Home Page
- Aparna Google Scholar
- Aparna DBLP
- Aparna ResearchGate
- Aparna LinkedIn
- Explore my research at Research with Montclair
- Explore my research at Research with New Jersey
- Best Paper Award - IEEE IISA Conference 2024, Crete, Greece
- Best Paper Award, Data Science/IoT Track, Springer's Intl. IoT Electronics & Mechatronics Conf 2024, Imperial College, London
- 1st Prize at RAISE-2024 Data Science National Competition for Montclair Team Machine Minds, Rutgers University, NJ
- Best Poster Award, New Jersey Big Data Alliance (NJBDA) Symposium, 2024, Rutgers University, NJ
- Best Paper Award, Robotics Track, IEEE IEMTRONICS 2020 (Online)
- Best Paper Award, IoT Track, IEEE UEMCON 2019, Columbia University, NY
- Best Short Paper Award, IT Track, IEEE IEMTRONICS, 2021 (Online)
- Best Graduate Presentation Award, Montclair Annual Research Symposium, April 2017, NJ
- Mention in NJ Newspaper for discussing Robotics and "Wave of the Future", Jun 2018
- Best Paper Award, Big Data Track, IEEE UEMCON 2016, Columbia University, NY
- Meeting Nobel Laureate, Turing Award Winners, et al. at AAAI-2020 conference, New York City, NY
- Meeting Turing Award Winner at WWW-2023 conference, Austin, Texas
- Meeting Turing Award Winner at WWW 2018 conference, Lyon, France
- Meeting Lithuanian Prime Minister Ingrida Simonyte at ECML-PKDD 2024 conference. Vilnius, Lithuania
- Research Tutorial Video on Commonsense Knowledge at ACM WSDM 2021 conference (Online)
- WWW-2023 Spotlight Mention Paper - Cultural Commonsense Knowledge
Research Projects
Decision Support in Green IT for Smart Environment and Sustainability
This multidisciplinary research in data mining and environmental management is supported by a grant from PSE&G. It involves investigating greener solutions for data centers with the goals of energy efficiency and adequate performance, thus falling in the paradigm of Green Information Technology. The role played by data mining techniques is significant here in the development of a decision support system GreenDSS to assist IT managers to head towards green computing in their respective data centers. Aspects addressed in this work include carbon footprint, power usage effectiveness, server sprawl, temperature, humidity, free cooling etc. This grant has supported a Ph.D. student Michael Pawlish in Environmental Management with Dr. Varde as the dissertation advisor in her capacity as Doctoral Faculty Member in that Program. It has led to publications in ACM's SIGMOD Record Journal, IJCAC journal, IEEE's ICIAFS, ACM's CIKM workshops, IEEE's ICDM workshops and various other multi-disciplinary venues. Further work emerging from this research on the use of cloud and hybrid models for green business solutions along with the DevOps (development and operations) paradigm appeared in ACM's SIGKDD Explorations journal. Results from this work have actually been used by PSE&G and Montclair State University for developing greener data centers. This work makes positive contributions on sustainability and smart environment to its green computing perspectives.
--------------------------------------------------
PhD Student: Michael Pawlish (Graduated: May 2014)
--------------------------------------------------
Co-authors: Dr. Stefan Robila (CS, Montclair), Dr. Anand Ranganathan (IBM Research)
--------------------------------------------------
Funding: PSEG Research Grant (2011 to 2013)
Explainable Terminology Evolution in Information Retrieval
This research in the overall area of Web and text mining started as joint work with Max Planck Institute, Germany during a research visit there. The goal of this project is to detect evolving terminology in responding to user queries on the Web by mining existing text archives. This is in order to enhance information retrieval by incorporating historical information on terms contained in queries. The methodology involves detecting semantically identical temporally altering concepts using the XAI (explainable AI) method of association rules, followed by suitable time-aware query translation over text archives. This is conducted in order to respond to user queries in a more pertinent manner, via incorporating semantics by detecting evolving terminology. This led to a Masters' Project by a CS graduate student Debjani Roychoudhury and a Masters' Thesis by a CS graduate student Amal Kalurachchi. It has been published in AAAI, ACM's EDBT and ACM's CIKM conferences.
------------------------------------------
M.S. Thesis Student: Amal Kaluarachchi (Graduated: May 2010)
------------------------------------------
M.S. Project Student: Debjani Roychoudhury (Graduated: May 2009)
------------------------------------------
Research Visit: Max Planck Institute, Germany (2008) - Supervisor: Dr. Gerhard Weikum
Urban Policy and Commonsense Knowledge for Smart Cities with Smart Governance
This project involves knowledge discovery from urban policy through modeling and mining of ordinances or local laws considering factors such as frequency, duration, attention to issues in sessions etc. A very important aspect is finding relationships between ordinances and smart city characteristics incorporating common sense knowledge (CSK) to capture human judgment in the mapping. The objective is to provide feedback to urban agencies on how well they are managing the given urban region and how much that region heads towards being a smart city. Another part of this work focuses on opinion mining over public reactions to the respective ordinances as expressed on social media through tweets. This involves polarity classification based on various levels of sentiments. This sentiment analysis indicates the satisfaction of the common public regarding the ordinances in their respective urban region and provides further assessment on how close this region is to being a smart city. The involvement of the public through such means is itself an aspect of the smart government characteristic through greater transparency in governance. Challenges include dealing with natural language in ordinances and tweets in addition to informal grammar, acronyms etc. in tweets which also entails the use of CSK. The source of CSK in this research is a worldwide repository called WebChild developed at Max Planck Institute for Informatics (MPII), Germany. Early work on this project started in 2015 during a faculty research visit to MPII. This has so far been published in a Tech Report of MPII 2015, IEEE UEMCON 2017, W3C's WWW 2018, IEEE ICTAI 2018, IEEE Big Data 2018 and I3E 2020. More work is in progress.
------------------------------------
PhD Student: Xu Du (ongoing)
------------------------------------
M.S. Thesis Student: Manish Puri (Graduated May 2019)
-------------------------------------
B.S. Research Student: Matthew Kowalski (Graduated May 2021)
-------------------------------------
Co-authors on Papers: Dr. Gerard de Melo (HPI, Germany), Dr. Boxiang Dong (CS, Montclair), Dr. Robert Taylor (EAES, Montclair)
--------------------------------------
Research Visit: Max Planck Institute, Germany (2015) - Supervisor: Dr. Gerhard Weikum
Funding: DA from Environmental Management; RA from Computer Science
Articles, Collocations and Prepositions in L2 English Text with Explainable AI
This research in the area of text mining and computational linguistics. It involves the classification of article errors, correction of odd collocations and prediction of preposition usage in texts written by L2 (non-native) English speakers and in automated translation to English by machines. Article errors pertain to entering articles where not needed, omitting articles where needed and entering the wrong article. Odd collocations involve using incorrect combination of terms such as powerful tea when the user actually means strong tea. Preposition prediction involves suggesting an appropriate preposition in a sentence. This is useful in writing aids typically designed for ESL learners. Mining the concerned text and deploying machine learning techniques such as classification and ensemble learning play an important role here. Related publications include conference papers in AAAI's FLAIRS 2010, IEEE's ICICS 2013 and a journal paper in ACM SIGKDD Explorations journal 2015. A research tutorial in ACM CIKM 2017 encompassed the collocations component of this work. All the methods involved in this work are in the category of XAI, e.g. decision trees for error-tracing, and word-based similarity search for preposition prediction.
-----------------------------------
M.S. Thesis Student: Alan Varghese (Graduated May 2013)
-----------------------------------
M.S. Project Student: Aliva Pradhan (Graduated May 2011)
-----------------------------------
M.S. Project Student: Pooja Bhagat (Graduated May 2014)
-----------------------------------
Co-authors on Papers: Dr. Anna Feldman (Linguistics & CS, Montclair), Dr. Eileen Fitzpatrick (Linguistics, Montclair), Dr. Jing Peng (CS, Montclair)
------------------------------------
Funding: TA from Computer Science; RA from Startup Funds
Commonsense Reasoning in Implicit Requirements, Autonomous Vehicles, Object Detection
This work is in the general area of deploying common sense knowledge (CSK) in various aspects of smart cities. One aspect entails the use of CSK in the identification and management of implicit requirements (IMRs) during the requirements specification phase in Software Engineering. As opposed to explicit requirements clearly outlined by users, implicit ones are more subtle and needed to be inferred to ensure the success of software development. A framework integrating CSK, Ontology and Text Mining is built in this research to address implicit requirements. This framework would be useful in implementing smart city tools by identifying IMRs from a user perspective. This work has been published in IEEE Big Data 2019 with more publications ongoing. Yet another aspect of this work deals with the deployment of CSK in the smart mobility characteristic of smart cities. More specifically, it involves embedding CSK in autonomous vehicles to enable them make more well-informed decisions analogous to excellent human drivers. This work aims to enhance automated driving especially with reference to safety related issues. It also involves augmenting object detection with commonsense knowledge, especially with the potential of being usable in autonomous vehicles. An important paradigm in this work is the deployment of commonsense knowledge for enhancing object detection. Some of our work here involves generating adversarial images for serving for object detection incorporating CSK, especially spatial commonsense. This work has been published in the IEEE ICTAI 2017, IEEE ICTAI 2018 and AAAI 2020. Much of this work has emerged from a project on commonsense knowledge applications, particularly with respect to domain-specific knowledge bases, initiated during a faculty research visit to Max Planck Institute for Informatics, Germany in 2015.
------------------------------------
PhD Student: Onyeka Emebo (Visiting Fullbright Scholar 2015-2016)
------------------------------------
M.S. Project Student: Abidha Pandey (Graduated May 2019)
-----------------------------------
B.S. Honors Student: Priya Persaud (Graduated May 2017)
------------------------------------
B.S. Research Student: Alexandra Kunkel (Graduated May 2019)
-------------------------------------
Co-authors on Papers: Dr. Niket Tandon (Allen AI, WA), Dr. Daramola Olawande (CPUT, South Africa), Dr. Vaibhav Anu (CS, Montclair)
-------------------------------------
Research Visit: Max Planck Institute, Germany (2015) - Supervisor: Dr. Gerhard Weikum
Funding: Fulbright Scholarship Program (2016); NSF LSAMP program (2017); Summer Research Grant (2020)
App Development for Smart Living: HCI, IoT and Domain Knowledge
This project addresses the realm of ubiquitous computing for smart living. It focuses on knowledge dissemination via mobile applications (apps) and other user-friendly software tools. An important app developed here is an ordinance-tweet mining app to disseminate knowledge on urban policy from a smart city perspective. This knowledge is discovered by mining ordinances and tweets on urban policy, especially to fathom how well the concerned region adheres to smart city characteristics based on these policies and their public opinions. It focuses on the NYC region. Another app targets climatic parameters such as temperature, humidity and precipitation that are analyzed using climate modeling in environmental management. The resulting outputs that entail searching relevant data and making future predictions are disseminated by developing a hydro-climate data app. This focuses on the NJ Passaic region. Yet another app in this work is for precipitation in Sub-Saharan Africa, a region where rainfall can be scarce. This precipitation data app acquires information from climate models and provides useful dissemination of knowledge based on various geographic locations to help public agencies, farmers and local residents plan their living. In addition, a precipitation data visualization tool is developed in this project to enable expert as well as naive users to conduct interactive visualization and analysis of Sub-Saharan data using trend-lines, map customization, filters etc. One more app developed for Smart Living includes a food donation app that helps suppliers and consumers connect with each other in order to productively use excess food from restaurants, stores etc. for food shelters and other donation facilities. It thereby helps to solve two important problems namely hunger and food waste. Much of the work in this area in addition to making a contribution to Smart Living also has broader impacts the United Nations Sustainable Development Goals. This project entails the work of M.S. students in CS and IT. Publications from this work include papers in IEEE UEMCON 2019 and I3E 2020. More work is ongoing.
----------------------------------------
M.S. Project Student: Drashti Pathak (Graduated Aug 2019)
-----------------------------------------
M.S. Project Student: Christina Varghese (Graduated Dec 2019)
-----------------------------------------
M.S. Project Student: Sudha Shah (Graduated May 2020)
-----------------------------------------
M.S. Project Student: Divyadharshini Karthikeyan (Graduated Dec 2020)
-----------------------------------------
Co-authors on Papers: Dr. Clement Alo (EAES, Montclair), Dr. Vaibhav Anu (CS, Montclair)
-----------------------------------------
Funding: PSEG-ISS Grant (2016); TA from Computer Science
Commonsense Knowledge, Computer Vision, Robotics and Smart Manufacturing
This research focuses on AI, Computer Vision and Robotics, with the deployment of commonsense knowledge (CSK). Some of this entails human-robot collaboration (HRC) with emphasis on Smart Manufacturing in Smart Cities. It involves learning from CSK sources online and also developing knowledge bases (KBs) entailing fundamental CSK as applicable to several robotics tasks. It involves mathematical modeling, algorithm development and design of HRC tasks based on the CSK concepts along with experimentation via simulation studies as well as real robotics laboratory experiments. An important approach proposed here includes CSK-based-HRC for robot task planning and optimization in smart manufacturing pioneered by us and published at IEEE Big Data 2020 and IEEE IEMTRONICS 2020 conferences with a best paper award at the IEEE IEMTRONICS 2020 conference, and paper published in the IEEE TASE journal. Other important tasks in this effort include harnessing search methods with and without heuristics in the context of robotic motion planning, published in IntelliSys 2021. More exciting work includes harnessing spatial CSK via collocations such as near, above, below etc. for objects in images to develop adversarial datasets in object detection, published in AAAI 2020 as a poster paper, and later as a demo paper in EDBT workshops. This system is called CSK-SNIFFER. It has motivated the development of another system called CSK-Detector that uses commonsense knowledge from a KB called Dice on facets such as plausibility, typicality, remarkability, and salience in order to detect objects in images and categorize the latter w.r.t. domestic tasks, especially useful in multipurpose robotics. This has further motivated the design and implementation of yet another system called Robo-CSK-Organizer, that uses commonsense knowledge from the KB ConceptNet in order to organize objects in rooms based on task relevance. Both CSK-Detector and Robo-CSK-Organizer outperform the state-of-the-art models based purely on deep learning. Moreover, they make contributions to Sustainable AI and Explainable AI due to minimizing complexity in learning, and providing interpretable solutions. The work has been published in IEEE Big Data 2022, and led to a best paper award in IEMTRONICS 2024. More journal publications are ongoing.
--------------------------------------
M.S. Thesis Student: Rafael Hidalgo (Graduated Jan 2024)
-------------------------------------
M.S. Thesis Student: Irina Chernyavsky (Graduated Jan 2023)
-------------------------------------
M.S. Thesis Student: Christopher J. Conti (Graduated Jan 2021)
-------------------------------------
M.S. AI Course Student: Laura Paulino (Graduated May 2021)
-------------------------------------
M.S. AI Course Student: Correy Hannum (Graduated Jan 2021)
-------------------------------------
Co-author on Papers: Dr. Simon Razniewski (Max Planck Institute for Informatics); Dr. Niket Tandon (Allen AI, WA); Dr. Weitian Wang (CS, Montclair)
-------------------------------------
Funding: NSF MRI Grant (2021 to 2024); TA from Computer Science; Summer Research Grant (2020)
Machine Learning in Image Mining: Nanoscale, X-ray, Audiovisual, and Drone Data
This work involves machine learning and data mining techniques in the area of image analysis for knowledge discovery. Early work here has been funded by a grant from NSF REU and supports undergraduate students from the tri-state area during summers. The focus of this grant is in the area of image processing and significant work occurs in the area of learning from image data at the nanoscale level. It entails proposing and implementing techniques for image mining useful in domain-specific decision-making. There is real data from researchers in Nanotechnology with applications having broader impact in health informatics. For example, the results of the learning are useful in finding a cheaper material instead of a more expensive material to develop a human body implant, if both materials yield similar results as evident from image similarity search. Publications from this work include a paper in SPIE 2010 conference, a presentation in ACM CCSC 2010 conference, and a paper in ICML 2010 Workshops. Further work in this general area includes decision support in automated detection of COVID-19 based on analysis of chest X-ray images on COVID-positive, pneumonia-positive and normal / healthy cases. This entails the deployment of computer vision models based on convolutional neural networks (CNNs) via transfer learning along with data augmentation. This part of the work has been published in IEEE Big Data 2020, and in the Springer MTAP journal. Another interesting piece of work includes mining data on Tuberculosis via cough symptoms a non-invasive method (vs. invasive chest X-rays). The audio data on cough samples is mapped to visual data using Mel Spectrograms and Mel Frequency Cepstral Coefficients, and then subjected to analysis using CNN, VGG and other models. Surprisingly a simple 1D-CNN gives the best results, in line with the Occam's Razor principle of preferring simpler theories over complex ones. This is published in IEEE Big Data 2023, and has led to a best paper award in IEEE IISA 2024, as well as a best poster award in the NJBDA symposium. Other work in this area includes image analysis in the area of earth science. This involves analysis of LiDAR and Multispectral images captured from drone-collected data to analyze dune formation from various perspectives. Machine learning techniques such as unsupervised learning with clustering, supervised learning with classification (random forest, decision trees) and deep learning with CNNs are being explored here. The results are useful to support domain-specific decision-making in earth science and fall in the broad realm of geo-informatics. This part of the work is supported by a grant from NOAA NJ Sea Grants Commission.
--------------------------------------------------
Ph.D. Student (Committee): Shane Daiek (ongoing)
---------------------------------------------------
B.S. Research Student: Britney Gonzales-Moodie (Graduated Jan 2022)
----------------------------------------------------
M.S. Thesis Student: Jyoti Yadav (Graduated May 2024)
-----------------------------------------------------
M.S. Project Student: Divyadharshini Karthikeyan (Graduated Jan 2021)
-----------------------------------------
Summer Research Student: Gregory Roughton (Completed: July 2009)
-----------------------------------------
Summer Research Student: Daniel Jackowitz (Completed: July 2010)
-----------------------------------------
Collaborators / Co-authors: Dr. Stefan Robila (CS, Montclair), Dr. Jianyu Liang (Materials Science, WPI, MA); Dr. Jorge Lorenzo-Trueba (EAES, Montclair), Dr. Hao Liu (CS, Montclair), Dr. Lei Xie (CUNY Hunter and Weill Cornell Medicine)
-----------------------------------------
Funding: NOAA NJ Sea Grants Commission (Sep 2023 to Sep 2025); NSF REU Grant (2009 to 2011); TA from Computer Science;
Cloud Computing in Big Data and Social Media
This project focuses on research in cloud computing with emphasis on managing and mining big data. Besides a thorough investigation of existing methodologies, it addresses the design and implementation of novel techniques and the enhancement of existing approaches for big data management and mining on the cloud. The project involves exploratory research with cloud technologies such as Hadoop, Hive and Mahout for big data. Various real world data sets are used in the context of areas such as scientific data management. Predictive analysis on the cloud is also conducted deploying machine learning algorithms in Mahout with specific reference to text classification, recommender systems and decision support. This project also involves opinion mining over cloud-based social media such as Twitter, where results of sentiment analysis are useful in applications such as recommenders. This has led to publications in the NJBDA Symposium, ACM CIKM's CloudDB 2013 and IEEE ICDM's KDCloud 2013 in addition to a best paper award at IEEE UEMCON 2019. A research tutorial has been presented at the DASFAA 2015 conference based on some outcomes of this work and related work by other researchers in the area. Further work includes the design and implementation of a novel Cloud Security Posture Management tool to incorporate crucial aspects of security into the cloud architecture by leveraging NIST based standards and other published guidelines. This has been published in IEEE UEMCON 2023.
-----------------------------------------
M.S. Project Student: Klavdiya Hammond (Graduated May 2013)
-----------------------------------------
M.S. Project Student: Shireesha Chandra (Graduated May 2012)
-----------------------------------------
M.S. Project Student: Ketaki Gandhe (Graduated May 2015)
-----------------------------------------
Co-author: Dr. Jiayin Wang (CS, Montclair); Dr. Jiacheng Shang (CS, Montclair)
-----------------------------------------
Funding: TA from Computer Science; NSF MRI grant (2020 to 2023)
GIS, Urban Sprawl and Air Quality Issues in Geo-informatics
This research spans Geographic Information Systems (GIS), Urban Sprawl and Air Quality. This entails mining spatial and temporal data to predict urban sprawl. It employs association rules with domain knowledge to discover relationships between various sprawl causing parameters such as unemployment, traffic, demographics, pollution etc., the impact of such parameters on sprawl and vice versa. It also involves dealing with pollution issues for further analysis on its relationship with urban sprawl. This is with the goal of air quality assessment incorporating public health factors using EPA standards. Data mining on multi-city data worldwide is conducted using classical techniques of association rule mining, clustering and classification to discover various causes of pollution in urban areas and predict air quality based on the analysis. This work also includes a social media mining component wherein reactions expressed by the public on pollution causing factors and its related solution mechanisms are assessed. Important outcomes of this work are prototype tools for sprawl prediction and for air quality assessment. Some of this research entails the early dissertation work of the PhD student Xu Du. This work has been published in KDD 2014 Bloomberg track, ICDE 2016 workshops and other venues.
------------------------------------
PhD Student: Xu Du (Graduated Jan 2021)
------------------------------------
M.S. Project Student: Anita Pampoore-Thampi (Graduated May 2014)
------------------------------------
Funding: DA from Environmental Management Program; RA from CS / EAES
XML-based Markup Languages and Semantic Web Standards
This work constitutes the use of XML and other standards in Web development for various real world applications. It is partly supported by a SHIP grant through Roche and Merck to fund Honors students in BS degree programs. One aspect of this work involves XML-based markup languages and Cloud Computing in management of EHR (Electronic Health Records). It entails investigating the use of the medical markup language MML, which constitutes a DSML (Domain Specific Markup Language) for storing and exchanging health records, proposing techniques for knowledge discovery over such XML based standards and also investigating the use of cloud technology in storage, retrieval and knowledge discovery pertaining to healthcare taking into account issues such as cost-effectiveness, risk analysis and scalability. Another aspect of this work includes the use of RDF, OWL and SPARQL for meta knowledge extraction in an application pertaining to university systems, helpful in ubiquitous computing by using Semantic Web standards. This constitutes has been published in IEEE UEMCON 2016, with a best paper award in one of the tracks. Other related publications in this entire project include a paper in the IEEE ICDM 2011 conference in their KDCloud workshop and another one in IEEE's ICIAFS conference. Research tutorials that entail the DSML part of this work and related research in XML, the Hidden Web and the Semantic Web have been given in Springer's DASFAA 2009 and ACM's EDBT 2011 conferences.
------------------------------------
M.S. Project Student: Aliva Pradhan (Graduated May 2011)
------------------------------------
B.S. Honors Student: Jonathan Tancer (Graduated May 2012)
-------------------------------------
Funding: Science Honors Innovation Program (2010 to 2012); TA from Computer Science
Clean Energy, Offshore Wind, Energy Equity and Environmental Issues
This project involves work in conjunction with the Clean Energy and Sustainability Analytics Center (CESAC) at Montclair. It includes a theme of Offshore Wind Energy with a fellowship-grant funded by NJEDA (New Jersey Economic Development Authority). An interesting piece of research entails sentiment analysis and topic modeling on posts pertaining to offshore wind facilities because many people express their opinion on public policy, and their inputs are significant in line with citizen science and transparent governance. This is published in IEMTRONICS 2024. Another piece of work pertains to the adequate placement of wind turbines, remote sensing on GIS data, and making recommendations based on discovering knowledge by unsupervised learning with clustering and supervised learning with deep learning as well as explainable classifiers. Some part of this work is published in IEEE Big Data 2024. A related piece of work is on automated detection of power plants for classification into multiple types such as solar, wind etc. in order to assist power estimation and energy management. It entails a novel architecture of GIS, CNN and ViT thus getting the best of both worlds, namely domain-specific knowledge (in geoinformatics via spatial masks and remote sensing) as well deep learning models (via CNN and ViT). This has been published in IEEE Sensors and AAAI Bridge Program. Yet another very interesting piece of research is energy equity w.r.t demographics and infrastructure, as well as related analysis of climate variables, energy policy, socio-economic data etc. This part of the work forms the core of a PhD student's dissertation. It has been published in IEEE ICTAI 2023, ACM SIGWEB 2023, AAAI 2023 Workshops, IEEE IISA 2024, and AAAI 2025 Bridge Program. Further work is ongoing in all these areas with active involvement of CESAC.
------------------------------------
PhD Student: Sarahana Shrestha (ongoing)
------------------------------------
M.S. Thesis Student: Isabele Bittencourt (Graduated May 2024)
------------------------------------
M.S. Thesis Student: Cristian Noriega-Monsalve (ongoing)
------------------------------------
M.S. Thesis Student: Blessing-Austin Gabriel (ongoing)
-----------------------------------
Funding: NJEDA Wind Tech Fellowship (2023-2025); Johnson and Johnson Internship; DA from Environmental Management