Faculty and Student Research Group Win Best Paper at IEEE IISA 2024 Conference
Drs. Varde, Liu and Antoniou along with Data Science MS student Jyoti Yadav won for their work titled Audiovisual Multimodal Cough Data Analysis for Tuberculosis Detection
Posted in: Awards and Recognition, Conferences and Presentations, Data Science, Department Research
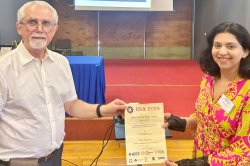
The project looks at the critical challenge of early detection of tuberculosis (TB). This research presents a novel multimodal approach utilizing audiovisual information from cough recordings to detect TB. We move beyond traditional image-based methods (such as sputum smear microscopy and chest X-rays) and examine the feasibility of leveraging cough recordings to differentiate TB cases. Two main audio processing techniques, namely Mel-Spectrograms and Mel-Frequency Cepstral Coefficients (MFCCs), are employed for feature encoding of audio recording in deep learning models for TB classification. Our proposed methods leverage a large challenge dataset comprising clinical data from over 1,105 participants and more than 502,252 cough recordings. Notably, a simple 1D Convolutional Neural Network (CNN) trained on MFCC features achieves an accuracy of 91%, surpassing the World Health Organization’s (WHO) requirements for TB screening tests. Our findings underscore the potential of MFCC features and 1D CNNs for accurate TB detection utilizing cough sound data. This approach adheres to the Occam’s Razor principle, which favors simpler models (such as 1D CNNs) when they yield comparable results. This research paves the way for further study in diverse populations and facilitates the development of accessible TB screening solutions, especially in resource-limited settings where only cough recordings are feasible, thereby emphasizing its notable real-world impacts.
The IISA conference series has become an international forum for researchers and professionals in all areas of Information, Intelligence, Systems and Applications. We invite submissions of papers presenting high-quality original research and developments for the conference tracks listed.