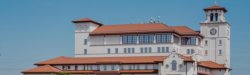
University Calendar
Brown Bags on Language, Information, and Computation: Sara Rosenthal (IBM)
Electronic Health Records (EHRs) contain both structured content and unstructured (text) content about a patient’s medical history. In the unstructured text parts, there are common sections such as Medical History, and Medications. These sections help physicians find information easily and can be used by an information retrieval system to return specific information sought by a user. It is common that the exact format of sections in a particular EHR does not adhere to known patterns. Being able to predict sections and headers in EHRs automatically is beneficial to physicians. Prior approaches in EHR section prediction have only used text data from EHRs and have required significant manual annotation. We used sections from medical literature (e.g., textbooks, journals, web content) that contain content similar to that found in EHR sections without the need of a time-consuming annotation effort. Our results show that medical literature can provide helpful supervision signal for this classification task.
Care management is a patient-centered approach to population health to assist patients and their support systems after being discharged from the hospital. In our work, we devised a novel annotation task evaluating a patient’s engagement with their health care regimen. The concept of engagement supplements the traditional concept of adherence with a focus on the patient’s affect, lifestyle choices, and health goal status. We created an engagement annotation task across two patient note domains: traditional clinical notes and a novel domain, care manager notes, where we find engagement to be more common. We trained two machine learning classifiers: an engagement model, and an efficient goal attainment model. The engagement model can successfully distinguish between engagement and lack of engagement in the unstructured notes. We found that incorporating engagement and other textual information from the unstructured notes significantly improves efficient goal attainment classification compared to using just structured information in the care management transaction records.
Bio
Sara Rosenthal is a Research Staff Member in the NLP group at IBM working on Question Answering. She spent the last few years working on NLP for HealthCare and Life Sciences. She is a co-organizer of the popular SemEval 2019 and 2020 Task, "OffensEval: Identifying and Categorizing Offensive Language in Social Media": https://sites.google.com/site/offensevalsharedtask/. Previously she was a co-organizer of the SemEval “Sentiment Analysis in Twitter" task that ran from 2013-2017. Prior to joining IBM, she completed her PhD in 2015 from Columbia University under the advisement of Dr. Kathleen McKeown. Her thesis was titled "Detecting Influencers in Social Media Discussions". This work included predicting demographics, opinion, agreement, persuasion, claims, and influencers in weblogs, micro-blogs, and discussion forums. Sara has served as an Area Chair for several *ACL conferences.