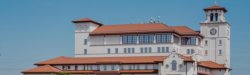
University Calendar
Dynamic Child Growth Prediction: A Comparative Methods Approach
Andrada Ivanescu, Montclair State University
Abstract
We introduce a class of dynamic regression models designed to predict the future of growth curves based on their historical dynamics. This class of models incorporates both baseline and time-dependent covariates, start with simple regression models and build up to dynamic function-on-function regressions. We compare the performance of the dynamic prediction models in a variety of signal-to-noise scenarios and provide practical solutions for model selection. We conclude that:
- prediction performance increases substantially when using the entire growth history relative to using only the last and first observation
- smoothing incorporated using functional regression approaches increases prediction performance
- the interpretation of model parameters is substantially improved using functional regression approaches.
Because many growth curve data sets exhibit missing and noisy data we propose a bootstrap of subjects approach to account for the variability associated with the missing data imputation and smoothing. Methods are motivated by and applied to the CONTENT data set, a study that collected monthly child growth data on 197 children from birth until month 15.